Lately, AI has become all the rage in cybersecurity. Smart new tools that detect sneaky malware or predict systems vulnerabilities seem to hit the market daily. Security ops teams worldwide race to integrate them into their defenses against the vast wilderness of threats they face.
It’s easy to get swept up in the excitement. After all, who wouldn’t want a digital guard dog that never sleeps, thinks faster than any human, and bolsters defenses tenfold?
But here’s the catch – many of these AI systems remain complete “black boxes” to us. Sure, the developers know what data they are feeding it – and yes, great insights or actions come out the other end a result. But the inner workings? Even the people who build these very tools struggle to decipher how (or why) the AI arrives at the decision it comes to – and that presents a number of technical and philosophical challenges.
So in our headlong rush to adopt AI, an awkward question gives us pause: Can we truly trust tools if we don’t understand how they arrive at their results? This emerging blindspot poses real food for thought in the fast-moving world of AI security today.
Peering into the Black Box
When experts describe AI systems as “black boxes,” what exactly does that mean? Well, it’s all about the inherent secrecy behind many popular AI approaches nowadays, especially those leveraging deep learning.
These systems can churn out incredibly impressive (and usually accurate) results, yet how they arrive at them remains wrapped in mystery. They employ elaborate algorithms and sprawling neural networks so complex that even their creators struggle to explain precisely why the AI makes the choices it does.
Get exclusive access to all things tech-savvy, and be the first to receive
the latest updates directly in your inbox.
Consider a deep learning system trained to detect malicious software lurking within vast mounds of code. It might pinpoint threats no human would ever notice by recognizing subtle patterns across millions of data points. But good luck asking why it flagged something as dangerous or how it makes those complex connections. Those answers are sealed away inside layers upon layers of computational “thinking” no human can readily penetrate.
In a way, this is similar to how the human brain operates. Each small decision is based upon many thousands (or even millions) of other decisions and variables, and pinpointing the exact “why” behind any given choice is almost impossible to pinpoint – at least not without mountains upon mountains of nuance.
That’s why finding ways to peer inside these black boxes represents such a crucial challenge. Doing so will allow us to put necessary guardrails on our AI tools before we embrace them whole-hog to protect our ever-more-digital world.
Why the Black Box Matters in Cybersecurity
When it comes to cybersecurity, it’s hard to overstate the stakes. A single misstep by an AI safeguarding a system could kick off a domino fall of troubles.
Take an AI threat detector. It seems great – until it incorrectly flags critical infrastructure as compromised. Cue major disruptive false alarms. Or worse, it misses a real attack, leaving security architecture wide open for data theft.
If we’re handing off serious decisions to AI, being extremely confident it can judge complex situations soundly is a must. But how can we have that faith if even the developers struggle to explain the thinking behind the AI’s calls?
The accountability questions get a bit convoluted too. Say an AI security tool with automation capabilities makes a choice that sparks a considerable breach that severely impacts millions of users. Do we blame the developers who couldn’t see how their creation operated below the surface? If not them, then who? With AI taking on more independent responsibility, vague issues around who answers for mistakes become urgent to solve.
Explainable AI (XAI) – Shedding Light on the Darkness
Fortunately, this troubling black box issue hasn’t gone unnoticed. Research fields like XAI – Explainable Artificial Intelligence creates tools and techniques to decipher what drives an AI system’s decision-making, dragging their inner workings kicking and screaming into the light. Some examples of burgeoning XAI approaches:
- Local Interpretable Model-Agnostic Explanations (LIME) – LIME attempts to simplify complex models. It tries to break down their local behaviors into easier-to-grasp explanations that anyone can understand.
- SHapley Additive exPlanations (SHAP) – SHAP is about assigning each feature within a model a value for how much it influences the prediction. This makes it clear which exact pieces impact the end result the most.
- Attention Mechanisms – These mechanisms basically spotlight what input data the AI pays the most attention to when making a decision. They illuminate what connections exist between various data points and conclusions the AI draw
Other Mitigation Approaches
At the same time, researchers also explore supplementary ways to eradicate black box secrecy, like:
- Simpler Models – Constructing models from square one for interpretability
- Human-in-the-Loop – Combining AI and human insight cooperatively.
- Transparency – External pressure for AI developers to open models for outside scrutiny
So while AI black boxes pose serious challenges now, brilliant minds tackling them ensure the future looks bright. We inch closer to a world where AI enhances security architecture while remaining open and accountable – not obscured behind veils of complexity and mystery.
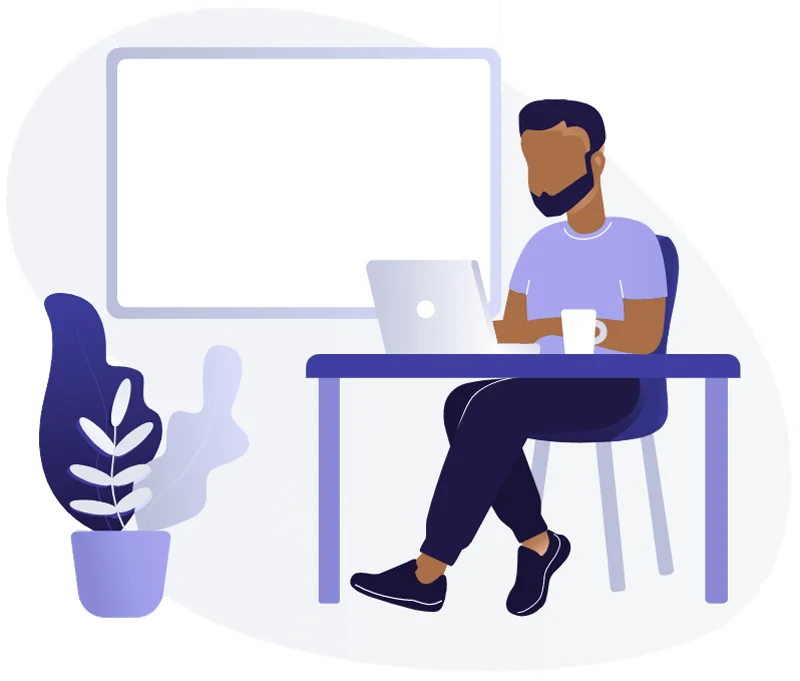
The Path Forward
The black box issue is certainly worth paying attention to when using AI for something as crucial as cybersecurity. But we’re starting to pry open these opaque systems to shed some light inside. Fields like XAI, along with approaches such as LIME, SHAP, and attention mechanisms, help make sense of how AI comes to its decisions. Other handy tactics like opting for simpler models, having humans double-check things, and pressuring developers to open up also move the needle.
The bottom line is that as AI capabilities explode, interpretability and accountability need to be priorities. We have to be totally confident about handing security of our ever-more-complex systems off to autonomous tools. Leaving gaps in understanding how our AI defenders operate is unlikely to lead to many favorable outcomes in the long run.